Identifying Recurring Patterns with Deep Neural Networks for Natural Image Denoising
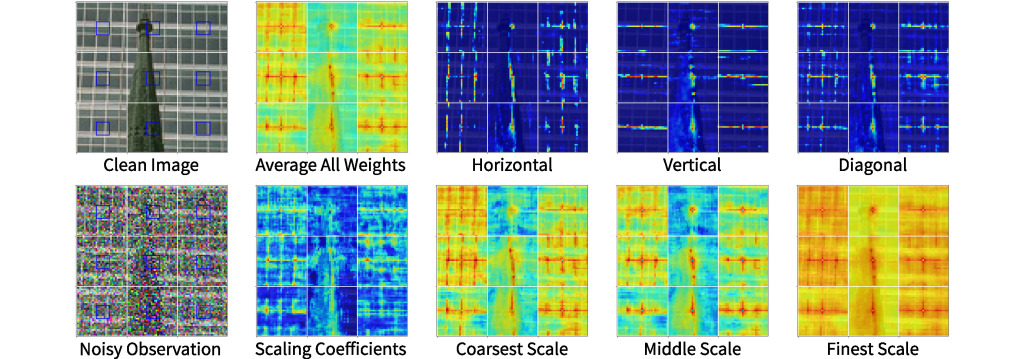
Image denoising methods must effectively model, implicitly or explicitly, the vast diversity of patterns and textures that occur in natural images. This is challenging, even for modern methods that leverage deep neural networks trained to regress to clean images from noisy inputs. One recourse is to rely on "internal" image statistics, by searching for similar patterns within the input image itself. In this work, we propose a new method for natural image denoising that trains a deep neural network to determine whether patches in a noisy image input share common underlying patterns. Given a pair of noisy patches, our network predicts whether different sub-band coefficients of the original noise-free patches are similar. The denoising algorithm then aggregates matched coefficients to obtain an initial estimate of the clean image. Finally, this estimate is provided as input, along with the original noisy image, to a standard regression-based denoising network. Experiments show that our method achieves state-of-the-art color image denoising performance, including with a blind version that trains a common model for a range of noise levels, and does not require knowledge of level of noise in an input image. Our approach also has a distinct advantage when training with limited amounts of training data.
Publication | [Paper] [Supplement] | WACV 2020 |
Downloads | Code & Models | GitHub |
This work was supported by the National Science Foundation under award no. IIS-1820693. Any opinions, findings, and conclusions or recommendations expressed in this material are those of the authors, and do not necessarily reflect the views of the National Science Foundation.
This site uses Google Analytics for visitor stats, which collects and processes visitor data and sets/reads cookies as described here.