Backprop with Approximate Activations for Memory-efficient Network Training
Ayan Chakrabarti Benjamin Moseley
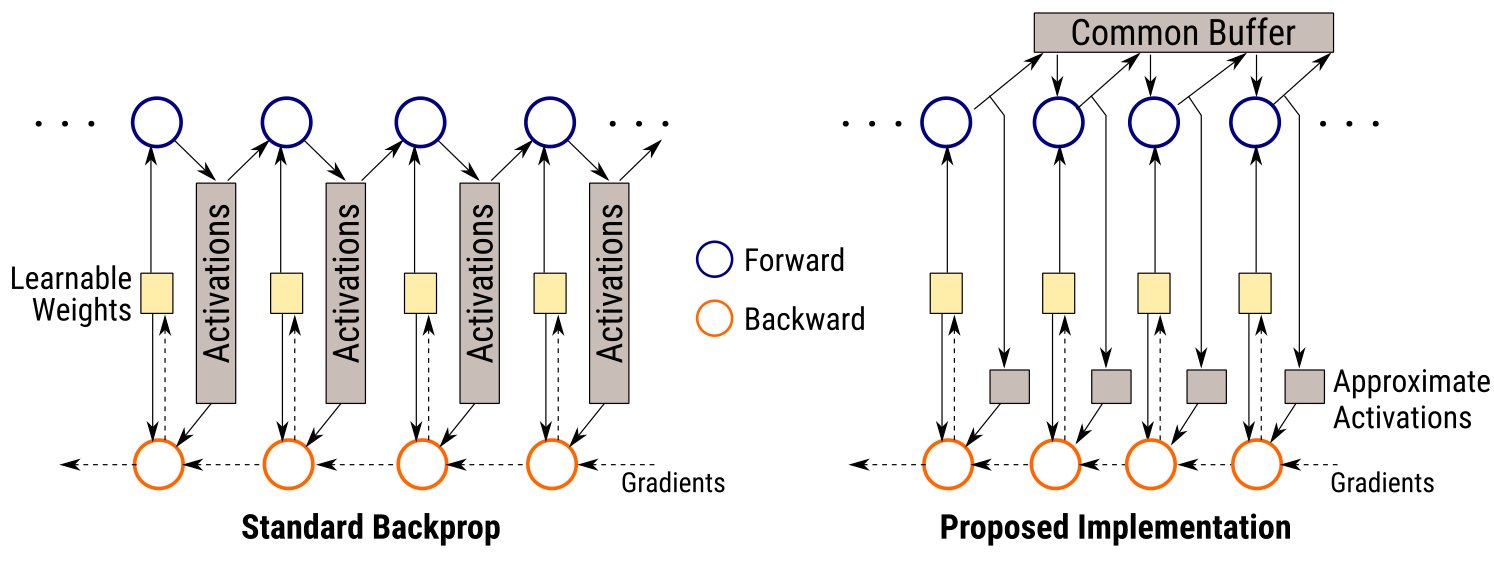
Training convolutional neural network models is memory intensive since back-propagation requires storing activations of all intermediate layers. This presents a practical concern when seeking to deploy very deep architectures in production, especially when models need to be frequently re-trained on updated datasets. In this paper, we propose a new implementation for back-propagation that significantly reduces memory usage, by enabling the use of approximations with negligible computational cost and minimal effect on training performance. The algorithm reuses common buffers to temporarily store full activations and compute the forward pass exactly. It also stores approximate per-layer copies of activations, at significant memory savings, that are used in the backward pass. Compared to simply approximating activations within standard back-propagation, our method limits accumulation of errors across layers. This allows the use of much lower-precision approximations without affecting training accuracy. Experiments on CIFAR-10, CIFAR-100, and ImageNet show that our method yields performance close to exact training, while storing activations compactly with as low as 4-bit precision.
[arXiv preprint] | NeurIPS 2019 paper |
[Source Code] | GitHub repo |
This work was supported by the National Science Foundation under grants IIS-1820693, CCF-1830711, CCF-1824303, and CCF-1733873, and a Google Research Award to Benjamin Moseley. Any opinions, findings, and conclusions or recommendations expressed in this material are those of the authors, and do not necessarily reflect the views of the National Science Foundation.
This site uses Google Analytics for visitor stats, which collects and processes visitor data and sets/reads cookies as described here.